Success story
Successful Integration of EO-Based Observations and Machine Learning in Spring Peak Flow Forecasting
Climate; Monitor snow cover; Urban Areas; Local and regional planners; Transportation; Travel, Tourism and Leisure
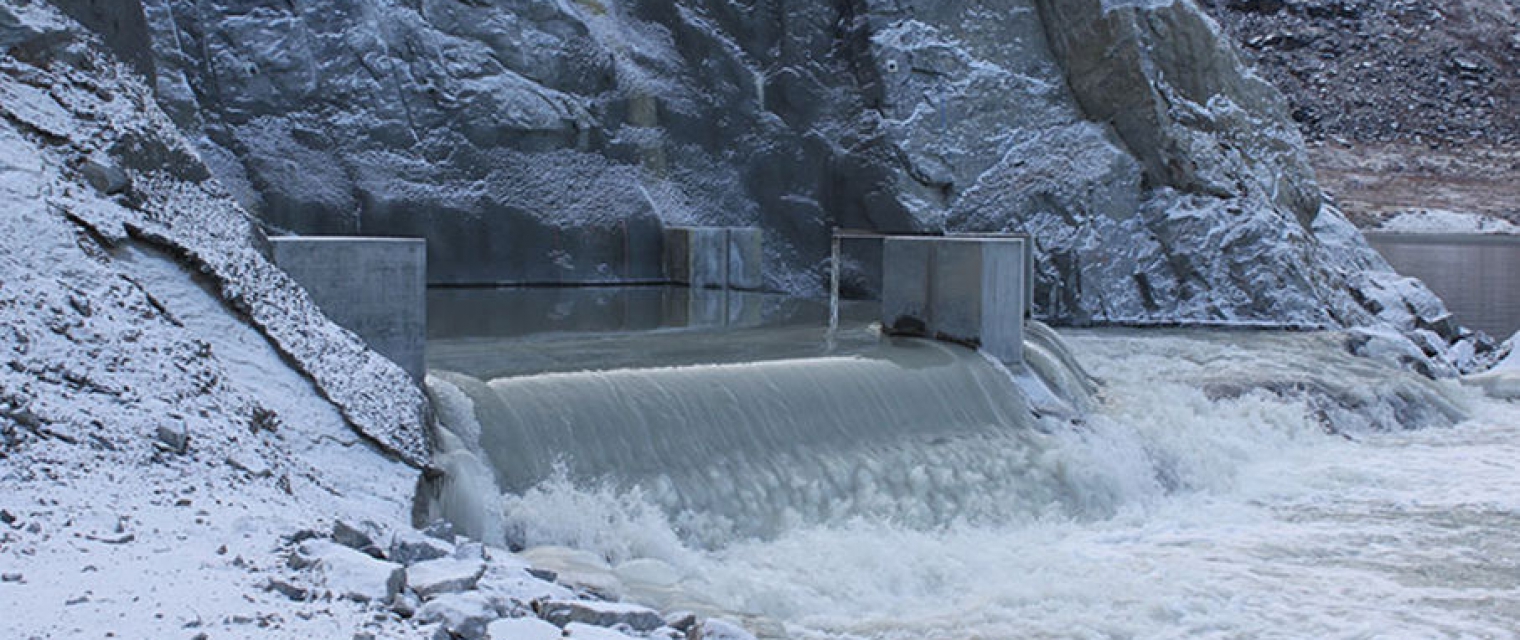
Profile
The Finnish Meteorological Institute (FMI) provides detailed information to support in more efficient hydropower operations through reduction of hydrological model uncertainties arising from incomplete information on snowpack states over large areas through increasing observation resolution and through data ingestion to the FMI HOPS model with both probability based and non-probabilistic frameworks. This service aims in reduction of spring snowmelt driven flood risks through more reliable hydrological nowcasts and forecasts. It is increasing forecast end-users' situational awareness and understanding of uncertainties and consequently providing a basis for optimization of hydropower operations. The outcome aims to bridge the gap between forecast providers and forecast end users by seeking solutions to remove barriers for information dissemination, application, and utilization.
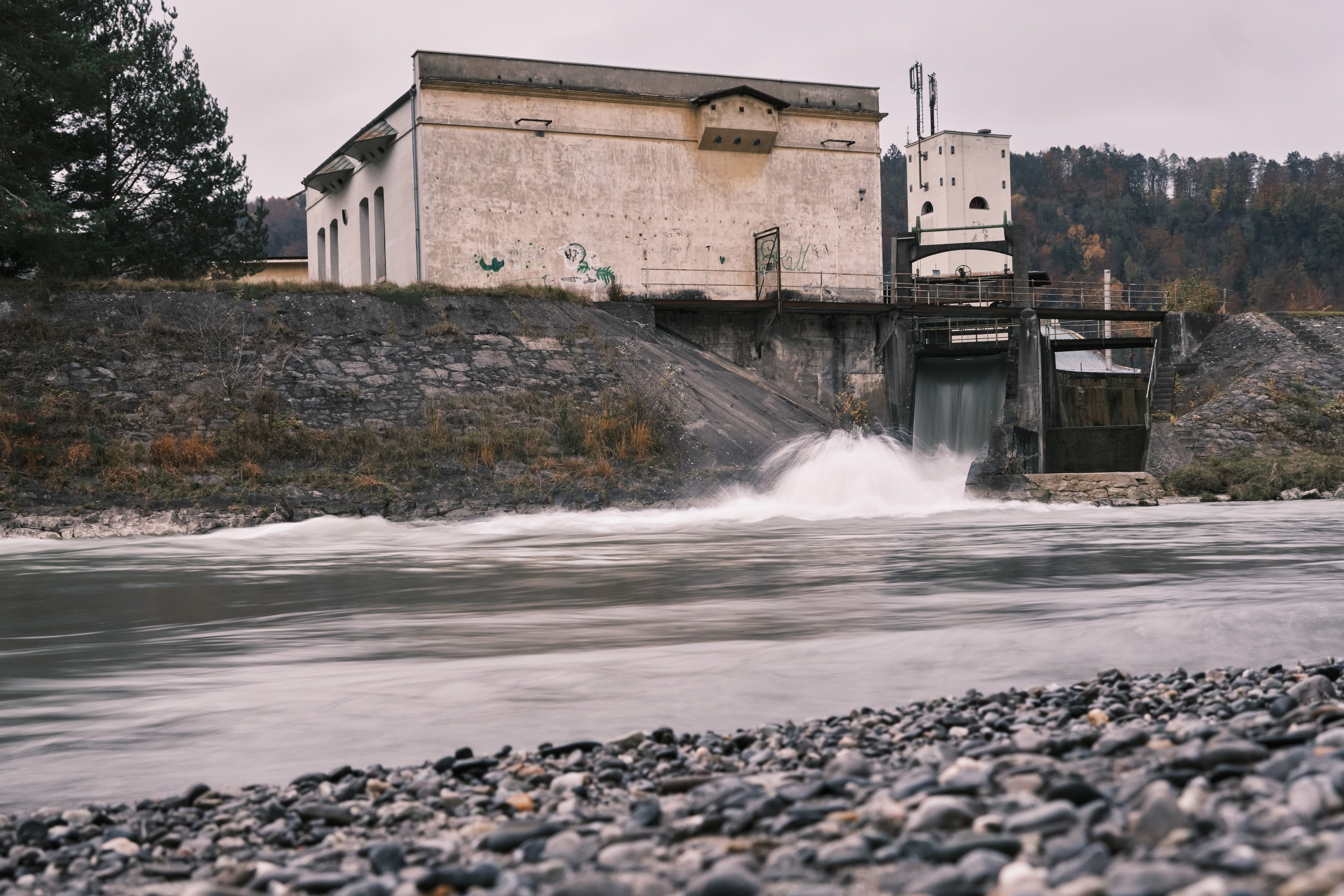
Proposal
The Finnish Meteorological Institute (FMI) provides detailed information to support in more efficient hydropower operations through reduction of hydrological model uncertainties arising from incomplete information on snowpack states over large areas through increasing observation resolution and through data ingestion to the FMI HOPS model with both probability based and non-probabilistic frameworks. This service aims in reduction of spring snowmelt driven flood risks through more reliable hydrological nowcasts and forecasts. It is increasing forecast end-users' situational awareness and understanding of uncertainties and consequently providing a basis for optimization of hydropower operations. The outcome aims to bridge the gap between forecast providers and forecast end users by seeking solutions to remove barriers for information dissemination, application, and utilization.
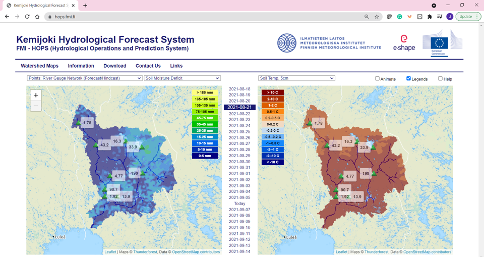
Benefits
- Successful assimilation of EO-based SWE data in improving spring snowmelt driven runoff peak timing and volume forecasts.
- Successful communication of uncertainties relating to long term seasonal ensemble forecasting.
- Successful implementation of machine learning based new streamflow forecasting methods.
- Successful integration of hydrometeorological data from multiple independent sources.
- Useful overall reduction of official forecast uncertainties through auxiliary information dissemination.
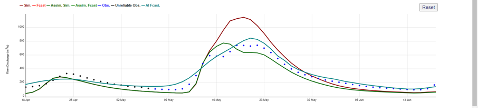